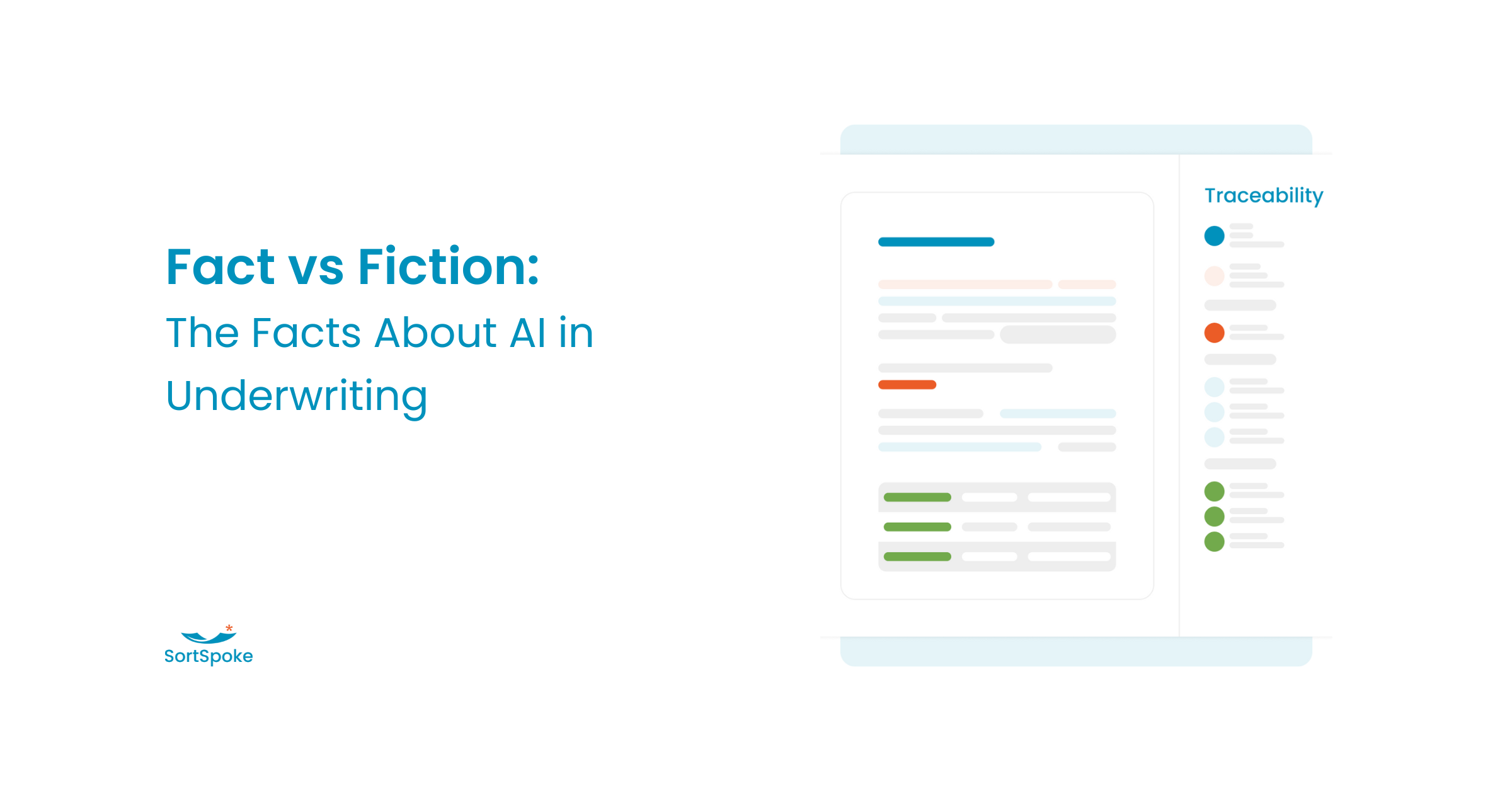
Beyond the Hype: The Facts About AI in Underwriting
TL;DR
- AI augments, not replaces: Successful insurance AI implementation enhances underwriters' capabilities, enabling them to process 5X more submissions while maintaining control and expertise.
- Human-in-the-loop validation: Effective AI systems in insurance combine automation with human oversight, ensuring accuracy, compliance, and continuous improvement.
- Strategic implementation: AI adoption should start with high-impact use cases like submission intake and document processing that deliver immediate ROI.
- Speed with accuracy: Properly implemented AI solutions dramatically reduce processing times while maintaining or improving quality through explainable, transparent systems.
- Integration flexibility: Modern AI solutions can integrate with existing systems with minimal IT lift, making implementation faster and more cost-effective than many insurers realize.
Insurance carriers face unprecedented challenges today. Increasing submission volumes, complex risk assessments, and customer expectations for rapid response times put immense pressure on underwriting teams. Meanwhile, the promise of artificial intelligence to transform these operations comes with its own concerns and misconceptions.
How do you separate AI reality from hype? What does successful implementation actually look like? And most importantly, how can you ensure AI enhances rather than disrupts your insurance operations?
This comprehensive guide addresses these questions head-on, providing insurance leaders with a balanced, practical roadmap for AI implementation. Drawing from real-world experiences and lessons learned, we'll move beyond common misconceptions to explore how AI can tangibly improve insurance operations while keeping humans at the center of the process.
Separating Fact from Fiction in Insurance AI
FICTON:
"AI Will Replace Human Underwriters"
In our work with dozens of insurance carriers and MGAs, we've consistently found that AI works best when designed to complement human expertise, not replace it. Successful implementations focus on automating the tedious, time-consuming aspects of document processing and data extraction—tasks that don't fully utilize underwriters' expertise.
Take submission intake, for example. Traditional approaches require underwriters to manually extract information from PDFs, spreadsheets, and emails before they can even begin their analysis. This process can consume 30-40% of an underwriter's time—time better spent on risk assessment and relationship building.
By implementing AI-powered document processing, one large P&C carrier was able to reduce manual data entry by 80%, allowing underwriters to focus on analyzing risks and making decisions. The result wasn't staff reduction—it was processing 5X more submissions with the same team, leading to significant growth in written premium.
FICTON:
"AI Implementation Is Prohibitively Expensive"
The perception that AI requires massive upfront investment stems from the early days of AI adoption and misunderstandings about implementation approaches. Today's reality is quite different:
- Specialized solutions vs. general AI: Purpose-built AI for insurance applications like document processing is far more cost-effective than building general-purpose AI capabilities.
- SaaS delivery models: Cloud-based, subscription approaches eliminate hefty upfront costs and infrastructure requirements.
- Rapid deployment: Modern, specialized AI solutions can be operational within days or weeks, not months.
- Minimal IT requirements: Solutions designed specifically for insurance can be implemented with limited IT resources.
"We initially hesitated on AI document processing because we assumed it would require a six-figure investment and months of IT work. The reality was deployment within three weeks and ROI within the first quarter. The key was finding a solution built specifically for insurance underwriting workflows." Head of Underwriting Operations, Mid-Size Specialty Insurer
When evaluating costs, the most important consideration is ROI timeframe. A properly implemented AI solution for document processing typically pays for itself within 3-6 months through:
- Reduced manual processing time
- Increased submission throughput
- Improved quote turnaround times
- Higher bind ratios due to faster response
- Reduced error rates and rework
FICTON:
"AI Complicates Regulatory Compliance"
Regulatory concerns are legitimate when it comes to AI implementation. However, the right approach to AI can actually strengthen compliance rather than undermine it. The key is implementing AI systems that prioritize:
- Explainability: Every AI decision or recommendation should be traceable to its source
- Transparency: The logic behind AI outputs should be clear and understandable
- Human oversight: Qualified professionals should review and have authority over AI suggestions
- Auditability: All decisions and data should maintain a clear audit trail
What is Explainable AI?
Explainable AI refers to systems designed to provide a clear understanding of how and why AI reaches specific conclusions. In insurance document processing, this means tracing extracted data points back to their original source in documents, making the AI's decision-making process transparent and auditable.
Insurance organizations subject to regulatory requirements like NYDFS Circular Letter No. 1 on AI and NAIC's AI principles should evaluate AI solutions based on their ability to provide:
- Documentation of data sources: Where information was extracted from and how it was processed
- Consistency in decision-making: Standard approaches to similar scenarios
- Traceable reasoning: Clear explanation for why particular data was extracted or flagged
- Ongoing validation: Regular testing and verification of outputs
Human-in-the-loop systems provide a crucial compliance advantage by ensuring qualified professionals validate AI-extracted information before it impacts underwriting decisions.
FICTON:
"AI Operates Without Human Oversight"
Perhaps the most persistent misconception about AI is that it operates as a "black box" making decisions without human input or oversight. This misunderstanding stems from early AI applications and science fiction rather than reality. Today's most effective insurance AI implementations use human-in-the-loop methodologies that:
- Preserve human judgment: AI makes suggestions that humans can accept, modify, or reject
- Learn from feedback: Systems improve based on human corrections
- Focus on confidence: AI identifies certainty levels and routes uncertain cases to human experts
- Enhance rather than replace: Technology handles repetitive tasks while humans make critical decisions
The typical workflow in a human-in-the-loop system:
- AI processes incoming submission documents, extracting key data points
- The system identifies confidence levels for each extraction
- High-confidence data is presented to underwriters for rapid verification
- Low-confidence extractions are flagged for closer human review
- Underwriters provide corrections that improve the system's future performance
- The verified data populates underwriting systems without manual re-entry
This approach combines the speed and consistency of AI with the irreplaceable judgment of experienced underwriters. The result is dramatically faster processing while maintaining or improving accuracy.
How to Suceed in 2025 - Practical Implementation Roadmap
Debunking the myths is just the beginning. To help you succeed where others struggle, here's a roadmap to follow to AI success in 2025.
Successful AI implementation in insurance requires a strategic approach focused on high-impact use cases, proper technology selection, and effective change management. Here's a practical roadmap based on real-world insurance implementation experiences:
1. Assessment Phase
The foundation of successful AI implementation begins with thorough assessment focused on identifying the right opportunities:
- Document the current process: Map your existing submission and underwriting workflows, identifying bottlenecks and manual touchpoints
- Quantify the opportunity: Calculate time spent on manual data extraction, document classification, and rework due to errors
- Analyze document types: Assess the variety and complexity of documents handled (applications, loss runs, financial statements, SOVs)
- Identify integration points: Document which systems currently receive extracted data (policy admin, rating engines, etc.)
High-impact starting points typically include:
- Submission intake and data extraction
- Schedule/location validation and normalization
- Statement of Value (SOV) processing
- Loss run analysis
- Financial statement extraction
2. Technology Selection
When evaluating AI solutions for insurance document processing, consider these key criteria:
Evaluation Criteria | What to Look For |
---|---|
Insurance-Specific Capabilities | Pre-built extractors for insurance documents (ACORD forms, loss runs, SOVs, etc.) |
Human-in-the-Loop Design | Intuitive interfaces for human validation and oversight of AI-extracted data |
Explainability | Clear traceability of extracted data to source documents |
Integration Flexibility | API capabilities, pre-built connectors to common insurance platforms |
Adaptation Capabilities | Ability to learn from human feedback and improve over time |
Implementation Timeline | Rapid deployment capabilities (days/weeks vs. months) |
IT Requirements | Minimal IT resources needed for implementation and maintenance |
The "build vs. buy" decision should consider both immediate needs and long-term strategy. Most insurers find that purpose-built solutions offer faster time-to-value than custom development, particularly for document processing and data extraction.
3. Implementation Best Practices
Successful AI implementations follow these proven practices:
Phased Approach
- Pilot phase: Start with a limited document set and small user group to validate the approach
- Targeted expansion: Incrementally add document types based on volume and impact
- Process integration: Connect AI outputs to downstream systems to eliminate manual re-entry
- Workflow optimization: Refine processes based on initial results and feedback
Change Management
AI implementation isn't just a technology project—it's a change management initiative. Successful adoption requires:
- Early stakeholder involvement: Include underwriters in the selection and implementation process
- Clear communication: Emphasize how AI will enhance rather than replace human roles
- Hands-on training: Provide interactive sessions demonstrating real-world use cases
- Success metrics: Establish and track clear KPIs that matter to end users
- Feedback mechanisms: Create channels for ongoing input and improvement suggestions
4. Measuring Success
Effective AI implementation requires clear, measurable objectives. Key performance indicators should include:
KPI Category | Metrics to Track |
---|---|
Efficiency Gains | - Time per submission - Submissions processed per underwriter - Straight-through processing rate |
Quality Improvements | - Data accuracy rate - Error reduction percentage - Rework frequency |
Business Impact | - Quote turnaround time - Submission-to-quote ratio - Quote-to-bind ratio - Premium written per underwriter |
User Adoption | - System usage metrics - User satisfaction scores - Feature utilization rates |
Navigating Common Implementation Challenges
Even well-planned AI implementations encounter challenges. Here's how to address the most common obstacles:
1. Data Quality and Accessibility
Document processing in insurance often involves inconsistent, incomplete, or poorly structured information. Successful approaches include:
- AI designed for imperfect data: Implement solutions that can handle real-world document variations
- Progressive improvement: Start with existing documents while establishing better standards for future submissions
- Targeted human review: Direct human attention to complex or low-confidence extractions
- Feedback loops: Use human corrections to continuously improve system performance
2. Legacy System Integration
Most insurers operate with complex technology ecosystems that have evolved over decades. Integration approaches that work include:
- API-first solutions: Select AI platforms with robust API capabilities for flexible integration
- Direct integrations: Leverage pre-built connectors to common insurance platforms
- Export flexibility: Ensure support for various data export formats (JSON, XML, CSV)
- Stepwise integration: Begin with simple data handoffs and progress to deeper system integration
"We initially worried about integrating AI with our 15-year-old policy admin system. The key was finding a solution that offered both modern APIs and traditional file-based integration methods, allowing us to implement quickly while planning our longer-term architecture." CIO, Regional Commercial Insurer
3. Talent and Expertise Gaps
AI implementation doesn't require turning your insurance professionals into data scientists. Practical approaches include:
- Solutions designed for business users: Select technologies built for insurance professionals, not technical experts
- Implementation partnerships: Work with vendors that provide implementation support and knowledge transfer
- Focused training: Develop role-specific training that emphasizes practical usage
- Internal champions: Identify and empower early adopters to support their peers
Responsible AI Implementation
Ethical considerations should be central to any AI implementation in insurance. Key areas to address include:
1. Ethical Considerations
- Explainable outcomes: Ensure all AI-driven decisions can be explained to stakeholders
- Bias detection: Regularly audit for unintended biases in data extraction and processing
- Human oversight: Maintain meaningful human review, especially for consequential decisions
- Transparency: Be clear with customers and regulators about how AI is used in your processes
2. Data Privacy and Security
Insurance document processing involves sensitive information that requires careful protection:
- Data minimization: Process only necessary information for the intended purpose
- Secure processing: Ensure end-to-end encryption and secure handling of all documents
- Compliance frameworks: Adhere to relevant regulations (GDPR, CCPA, NYDFS, etc.)
- Audit trails: Maintain comprehensive logs of all document access and processing
The Future of Insurance AI (2025-2030)
While focusing on practical implementation today, it's worth considering how insurance AI will evolve in the coming years:
Emerging Capabilities
- Multimodal understanding: AI that simultaneously processes text, tables, images, and diagrams within insurance documents
- Intelligent workflows: Adaptive processing paths based on document content and context
- Cross-document intelligence: Systems that analyze relationships between multiple submission documents
- Predictive document requests: AI that identifies missing information and automatically requests it from brokers
Evolving Use Cases
The most forward-thinking insurers are already exploring:
- Automated submission triage: AI-driven routing based on risk characteristics and underwriter expertise
- Integrated risk assessment: Combining extracted submission data with external information for comprehensive evaluation
- Dynamic capacity allocation: AI-supported decisions on optimal resource allocation across submission pipeline
- Proactive risk insights: Identifying emerging patterns across submission data to inform underwriting strategy
Conclusion
The successful implementation of AI in insurance isn't just about technology—it's about transformation that empowers underwriters to work more efficiently, effectively, and strategically. By focusing on human-in-the-loop approaches that combine the best of AI and human expertise, insurers can achieve the seemingly contradictory goals of processing more submissions while improving quality and compliance.
The most successful organizations will be those that move beyond common misconceptions to implement practical, responsible AI solutions that address real business challenges while keeping underwriters at the center of the process.
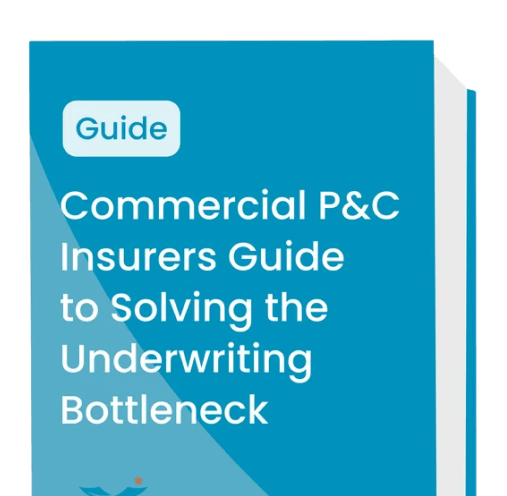